The CRM Audit - Part 2
Last updated on January 2, 2023 at 12:51 PM.Among the many digital audits that marketers perform to increase their ROI, the CRM audit is one of the most important and yet one of the least frequently performed analyses. Particularly valuable because its findings can effectively expand existing customer relationships, unfortunately quite rarely performed because this requires quite precise knowledge around the behavior of the target audience. In the second part of our two-part series, we will show you how to audit your CRM in seven steps. Let's go!
Step 1: Analysis of Data Sources
In most cases, the data in our CRM system comes from more than one source. On the digital side, data is obtained via forms in a wide variety of forms. A data record generated by newsletter subscription usually contains an email address. A whitepaper download, on the other hand, may contain more valuable information, such as the position in the company, the annual marketing budget, or the size of the company.
We have not generated every data set ourselves. Sometimes we have obtained it through a cooperation where we did not create the form ourselves. Individual characteristics of a dataset may also be enriched from third-party sources, such as commercial data enrichment services. Our user's journey may have started on a native advertising platform that provides us with attribution data, social media platforms provide us with important demographic data, and public platforms such as the Federal Gazette provide us with important company data. Not to be neglected is data obtained through physical contacts at trade shows, conventions or sales events. Before this data enters our system (if it does at all), important data from a business card may be misappropriated by the sales representative or several business cards may be entered in different forms.
Equally important is the method by which this data entered our system. A common form is extraction via API, FTP, CSV or Excel spreadsheet. Particular care should be taken with Excel spreadsheets as they include formatting information (UTF encoding, currency, date scheme, etc.).
Data can also be generated via website scraping. Since the front end of a website was not designed to be read by crawlers, this is also likely to produce incomplete results. Commercial providers therefore offer scraping including post-qualification of the data.
The users in our CRM system come from different sources, which in turn generate different characteristics of a data set. Depending on the campaign, it is important which characteristics are to be triggered. Therefore, we must always assume that the campaign segment is significantly smaller than the total number of users in our CRM system. In order to be able to target the largest possible number of addressees in our campaigns, we should not have to be limited by the incompleteness of a data set. We should always remember that any contact could become a customer.
Step 2: Unify Data for the Target System
Next, we need to unify the data we have collected across the various sources for our target system. There are no limits to the number of variants: Fields are in text format, but the target system processes only numeric data, some fields are limited by character length in the target system, contents of columns such as "Name" must be split into "First Name" and "Last Name", in multilingual systems umlauts are output as special characters, etc.
Step 3: clean up the errors from your data
Step 3: Clean Data
In this step we clean the data from content errors. We correct erroneous data on a content level. We distinguish between correction with business context and without business context. Typical errors are spelling mistakes, incorrect designations, invalid designations or data duplicates.
Step 4: Standardize Data
Even the most contextually correct data can be unusable. An example of this is the German country abbreviation DE, for which GER could also be used without further ado. Both abbreviations make the same statement, but we get two data points. Different systems have different data standards. We have to define a standard for ourselves before we can use the collected data in automation, analysis and reports.
Typical sources of errors are country abbreviations mentioned above, formats of phone numbers, abbreviations for company names, product names or all kinds of coding terms like DACH or EMEA.
The larger the number of records to be processed becomes, the more we have to think about how to scale the process. What at first appears to be a task for a spreadsheet in Excel must take place via rule-based automation when dealing with data sets in the seven-digit range. However, rule-based standardization also has its pitfalls: we have to get an overview of the wealth of variants of errors before we eliminate them by rule. To ensure that we are not limited by our own horizons, we should resort here to a technical solution in which rules are already stored.
Step 5: Correlate Data
We often combine data from different sources in order to be able to make additional statements about them. In order to establish these correlations and possibly enrich a data set, we need a unique characteristic for the correlation, a marker that we are dealing with one and the same person. This marker is the unique identifier. In a convenient case, it is a system ID that we can find in the various source systems. If such data is not available to us, we have to establish a connection with the combination of data. One of the most frequently used unique identifiers is the email address. However, even here there is already a higher error rate, since a person can be registered with both his private and his business email address. If email address, website domain, or company name are not available to us, we will get poor results.
Step 6: Segment Data
With segmentation, we group data based on common characteristics. These characteristics can be demographic, business, technical, and behavioral. Segmenting data is the basis for rule-based automation of processes. Segmentations used in a business context include:
- B2B demographics (function, role, level, buyer persona),
- B2B firmographics (industry, company size, revenue, location),
- B2B technographics (CMS, CRM, MAP, ERP),
- B2B behavior (email response wait time, preferred information materials, preferred communication channels).
- B2C demographics (age, gender, education level, location, income), and
- B2C behaviors (purchase history, mobile device usage, onsite history).
Step 7: Add Context
In this final step, we add additional context to the entries in our database to help us communicate with the individual. This includes additional industry classifications, classifications of a particular industry, maturity level of a company, and any other differentiations that can be applied and standardized in some way to our database. Further, we can use the records to add characteristics that describe the relationship between us and our customer, for example, "new customer," "existing customer," or "potential customer." We can contextualize customers based on our shared communication history, for example, first-time customer, inactive first-time customer, activated first-time customer, repeat customer, etc. There are no limits to the imagination.
Summary
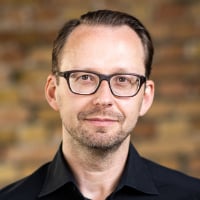
Gerrit Grunert is the founder and CEO of Crispy Content®. In 2019, he published his book "Methodical Content Marketing" published by Springer Gabler, as well as the series of online courses "Making Content." In his free time, Gerrit is a passionate guitar collector, likes reading books by Stefan Zweig, and listening to music from the day before yesterday.